Trust and quality key in AI debate
Latest think ahead events see experts discuss the limitations of data and training, and how they can be overcome
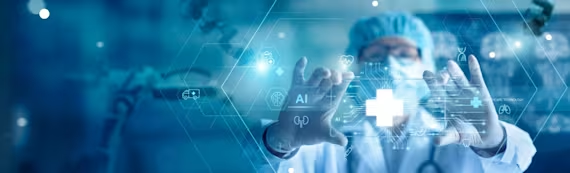
Artificial intelligence (AI) is only as good as the quality of data that informs it.
That was the observation of AI expert and London Business School (LBS) Sloan Fellow Barbara Domayne-Hayman at the latest LBS think ahead event, ‘How Will AI Innovation in Healthcare Improve Our Lives?’
The Francis Crick Institute’s entrepreneur-in-residence was joined on a panel by Andrew Vallance-Owen, Chief Medical Officer at Medicover AB, Nicos Savva, Professor of Management Science and Operations at LBS, and Crystal Ruff, Senior Director Neuroscience and Vaccines at Takeda. Julian Birkinshaw, Professor of Strategy and Entrepreneurship and Vice-Dean of LBS, moderated the session.
Dr Domayne-Hayman emphasised that health-related AI faced additional challenges related to human samples.
“A lot depends on how the sample was taken, how the sample was stored, how it was analysed – the quality of that,” she said.
“Then on top of the quality of that (data), you’ve then got the potential biases that are introduced by not having a representative sample of many, many different types. So, for instance, different ethnicities and also gender, because historically clinical trials have been done mainly on men of a certain age range, 18 to 60.”
Professor Savva echoed Dr Domayne-Hayman’s observations, but emphasised even imperfect data could be used if its limitations were understood.
“I think the right conclusion is that whenever we collect data, we need to be very careful in documenting the data collection, the data generation process and, if there are any imperfections, for these imperfections to be identified and to, at the very least, address it when we are training modes,” he said.
“(There are) very simple ways of addressing it, we will know the boundaries of the model: that the model is not very good at making predictions outside its training set or we might want to deploy some ways to overcome data limitations. But obviously all of this is impossible if we start with the assumption that the data is perfect.”
Dr Vallance-Owen pointed to practical ways trust could be built in healthcare AI, including greater data transparency.
“It’s been suggested that all models should clearly describe their data sources. That’s not done at the moment, it’s completely unknown as to where their data comes from. Of course, in many (instances) it comes from all sorts of places, which makes it difficult to do that.”
He said training should also focus on improving representation and reducing bias.
“The training should always try and make it more representative and less biased, and that’s a long-term thing to do because so much of research is North American and European and there is this bias.”
But reliable data also requires secure storage. Dr Ruff pointed to data security and how it could be combined and shared as a key risk in in the use of AI in healthcare settings that could not be ignored.
“I think we saw a great example in Covid, one of the silver linings of something very terrible, is a lot of pharma companies were able to collaborate, work together, to get to a vaccine as fast as possible,” she said. “One of the things that we’re looking at is: How do we share data? How do we access data? Even internally within pharma companies it’s so siloed. And so solutions have emerged that use what’s called federated data systems and sharing of data, whereby the data can stay in its silo, it can stay in its server or storage room … – one’s in China, one’s in Zurich and one is in Brazil - and it can still be stored with that country-level specific governance, but you can send queries on a network on top of that.”
The discussion attracted 600 viewers and follows an earlier think ahead event on ‘The Business Implications of AI’.
The next think ahead event ‘The Road to COP28 – Aligning Expectations with Actions’ will be 16 November 2023.